Abstract: Researchers have developed an AI-powered “digital tongue” able to distinguishing refined variations in liquids, reminiscent of milk freshness, soda varieties, and occasional blends. By analyzing sensor information by way of a neural community, the system achieved over 95% accuracy in figuring out liquid high quality, authenticity, and potential questions of safety. Apparently, when the AI was allowed to pick its personal evaluation parameters, it outperformed human-defined settings, exhibiting the way it holistically assessed refined information.
This know-how, which makes use of graphene-based sensors, might revolutionize meals security assessments and probably lengthen to medical diagnostics. The system’s AI insights additionally present a singular view into the neural community’s decision-making course of. This innovation guarantees sensible purposes throughout industries the place high quality and security are paramount.
Key Details
- The digital tongue makes use of AI to establish refined variations in meals high quality with over 95% accuracy.
- The system’s neural community performs higher when defining its personal evaluation metrics.
- Functions lengthen past meals security to potential use in medical diagnostics.
Supply: Penn State
A lately developed digital tongue is able to figuring out variations in comparable liquids, reminiscent of milk with various water content material; various merchandise, together with soda varieties and occasional blends; indicators of spoilage in fruit juices; and situations of meals security considerations.
The group, led by researchers at Penn State, additionally discovered that outcomes had been much more correct when synthetic intelligence (AI) used its personal evaluation parameters to interpret the information generated by the digital tongue.
The researchers printed their outcomes at this time (Oct. 9) in Nature.
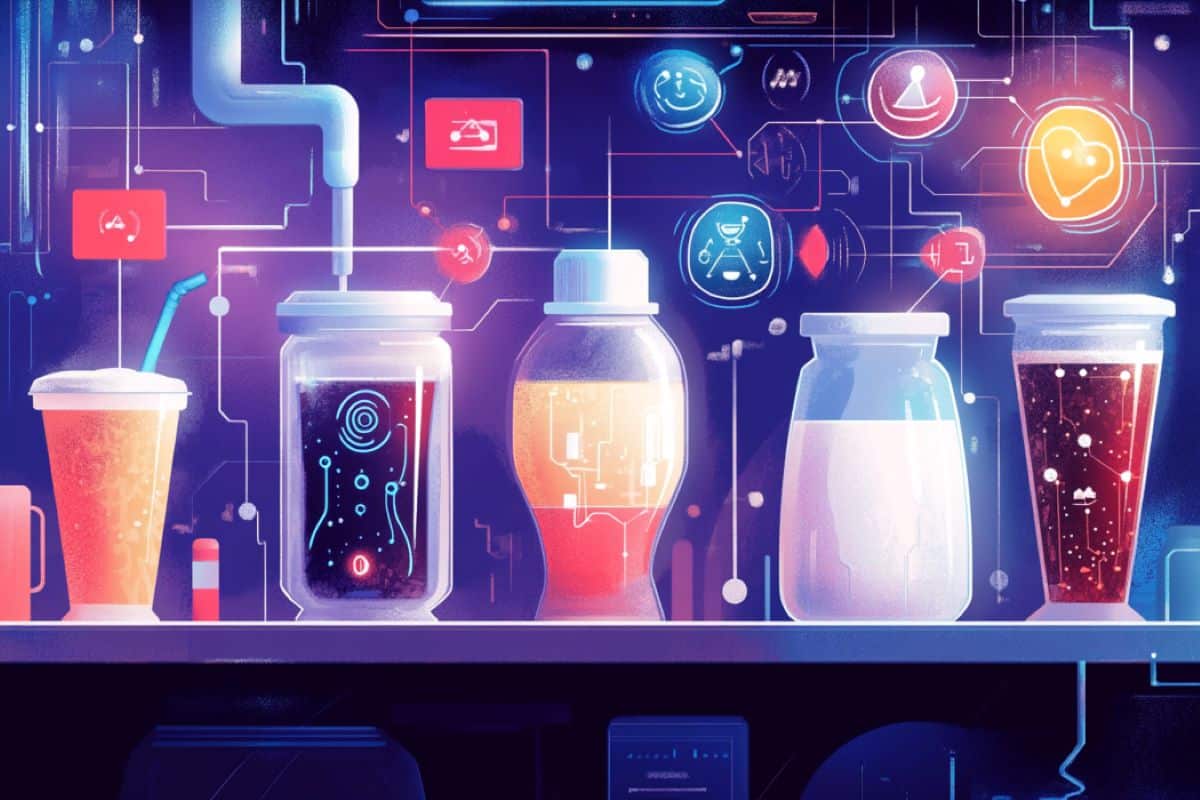
In keeping with the researchers, the digital tongue will be helpful for meals security and manufacturing, in addition to for medical diagnostics. The sensor and its AI can broadly detect and classify numerous substances whereas collectively assessing their respective high quality, authenticity and freshness.
This evaluation has additionally supplied the researchers with a view into how AI makes selections, which might result in higher AI growth and purposes, they stated.
“We’re attempting to make a synthetic tongue, however the strategy of how we expertise completely different meals includes extra than simply the tongue,” stated corresponding creator Saptarshi Das, Ackley Professor of Engineering and professor of engineering science and mechanics.
“We now have the tongue itself, consisting of style receptors that work together with meals species and ship their info to the gustatory cortex — a organic neural community.”
The gustatory cortex is the area of the mind that perceives and interprets numerous tastes past what will be sensed by style receptors, which primarily categorize meals by way of the 5 broad classes of candy, bitter, bitter, salty and savory. Because the mind learns the nuances of the tastes, it could higher differentiate the subtlety of flavors.
To artificially imitate the gustatory cortex, the researchers developed a neural community, which is a machine studying algorithm that mimics the human mind in assessing and understanding information.
“Beforehand, we investigated how the mind reacts to completely different tastes and mimicked this course of by integrating completely different 2D supplies to develop a type of blueprint as to how AI can course of info extra like a human being,” stated co-author Harikrishnan Ravichandran, a doctoral scholar in engineering science and mechanics suggested by Das.
“Now, on this work, we’re contemplating a number of chemical compounds to see if the sensors can precisely detect them, and moreover, whether or not they can detect minute variations between comparable meals and discern situations of meals security considerations.”
The tongue contains a graphene-based ion-sensitive field-effect transistor, or a conductive system that may detect chemical ions, linked to a synthetic neural community, educated on numerous datasets. Critically, Das famous, the sensors are non-functionalized, that means that one sensor can detect several types of chemical compounds, fairly than having a particular sensor devoted to every potential chemical.
The researchers supplied the neural community with 20 particular parameters to evaluate, all of that are associated to how a pattern liquid interacts with the sensor’s electrical properties. Primarily based on these researcher-specified parameters, the AI might precisely detect samples — together with watered-down milks, several types of sodas, blends of espresso and a number of fruit juices at a number of ranges of freshness — and report on their content material with higher than 80% accuracy in a few minute.
“After reaching an affordable accuracy with human-selected parameters, we determined to let the neural community outline its personal figures of benefit by offering it with the uncooked sensor information.
“We discovered that the neural community reached a close to splendid inference accuracy of greater than 95% when using the machine-derived figures of benefit fairly than those supplied by people,” stated co-author Andrew Pannone, a doctoral scholar in engineering science and mechanics suggested by Das.
“So, we used a way known as Shapley additive explanations, which permits us to ask the neural community what it was pondering after it decides.”
This method makes use of sport idea, a decision-making course of that considers the alternatives of others to foretell the result of a single participant, to assign values to the information into account.
With these explanations, the researchers might reverse engineer an understanding of how the neural community weighed numerous parts of the pattern to make a remaining willpower — giving the group a glimpse into the neural community’s decision-making course of, which has remained largely opaque within the area of AI, in keeping with the researchers.
They discovered that, as a substitute of merely assessing particular person human-assigned parameters, the neural community thought of the information it decided had been most vital collectively, with the Shapley additive explanations revealing how vital the neural community thought of every enter information.
The researchers defined that this evaluation might be in comparison with two folks ingesting milk. They will each establish that it’s milk, however one particular person might imagine it’s skim that has gone off whereas the opposite thinks it’s 2% that’s nonetheless recent. The nuances of why will not be simply defined even by the person making the evaluation.
“We discovered that the community checked out extra refined traits within the information — issues we, as people, wrestle to outline correctly,” Das stated.
“And since the neural community considers the sensor traits holistically, it mitigates variations that may happen day-to-day. When it comes to the milk, the neural community can decide the various water content material of the milk and, in that context, decide if any indicators of degradation are significant sufficient to be thought of a meals security difficulty.”
In keeping with Das, the tongue’s capabilities are restricted solely by the information on which it’s educated, that means that whereas the main focus of this examine was on meals evaluation, it might be utilized to medical diagnostics, too.
And whereas sensitivity is vital regardless of the place the sensor is utilized, their sensors’ robustness gives a path ahead for broad deployment in numerous industries, the researchers stated.
Das defined that the sensors don’t should be exactly equivalent as a result of machine studying algorithms can take a look at all info collectively and nonetheless produce the correct reply. This makes for a extra sensible — and cheaper — manufacturing course of.
“We discovered that we are able to reside with imperfection,” Das stated. “And that’s what nature is — it’s filled with imperfections, however it could nonetheless make sturdy selections, similar to our digital tongue.”
Das can also be affiliated with the Supplies Analysis Institute and the Departments of Electrical Engineering and of Supplies Science and Engineering. Different contributors from the Penn State Division of Engineering Science and Mechanics embody Aditya Raj, a analysis technologist on the time of the analysis; Sarbashis Das, a graduate scholar on the time of analysis who earned his doctorate in electrical engineering in Might; Ziheng Chen, a graduate scholar in engineering science and mechanics; and Collin A. Value, a graduate scholar who earned his bachelor of science in engineering science and mechanics in Might. Mahmooda Sultana, with the NASA Goddard Area Flight Middle, additionally contributed.
Funding: A Area Know-how Graduate Analysis Alternatives grant from NASA supported this work.
About this AI analysis information
Writer: Ashley WennersHerron
Supply: Penn State
Contact: Ashley WennersHerron – Penn State
Picture: The picture is credited to Neuroscience Information
Authentic Analysis: Closed entry.
“Strong chemical evaluation with graphene chemosensors and machine studying” by Saptarshi Das et al. Nature
Summary
Strong chemical evaluation with graphene chemosensors and machine studying
Ion-sensitive field-effect transistors (ISFETs) have emerged as indispensable instruments in chemosensing purposes.
ISFETs function by changing adjustments within the composition of chemical options into electrical alerts, making them splendid for environmental monitoring, healthcare diagnostics and industrial course of management. Current developments in ISFET know-how, together with functionalized multiplexed arrays and superior information analytics, have improved their efficiency.
Right here we illustrate some great benefits of incorporating machine studying algorithms to assemble predictive fashions utilizing the intensive datasets generated by ISFET sensors for each classification and quantification duties. This integration additionally sheds new mild on the working of ISFETs past what will be derived solely from human experience.
Moreover, it mitigates sensible challenges related to cycle-to-cycle, sensor-to-sensor and chip-to-chip variations, paving the way in which for the broader adoption of ISFETs in industrial purposes. Particularly, we use information generated by non-functionalized graphene-based ISFET arrays to coach synthetic neural networks that possess a exceptional skill to discern situations of meals fraud, meals spoilage and meals security considerations.
We anticipate that the fusion of compact, energy-efficient and reusable graphene-based ISFET know-how with sturdy machine studying algorithms holds the potential to revolutionize the detection of refined chemical and environmental adjustments, providing swift, data-driven insights relevant throughout a large spectrum of purposes.
Discussion about this post