Abstract: Researchers have developed a Genetic Development Rating (GPS) utilizing synthetic intelligence to foretell the development of autoimmune illnesses from preclinical signs to full illness. The GPS mannequin integrates genetic knowledge and digital well being data to offer personalised threat scores, enhancing prediction accuracy by 25% to 1,000% over current fashions.
This methodology identifies people at increased threat earlier, enabling well timed interventions and higher illness administration. The framework is also tailored to check different underrepresented illnesses, providing a breakthrough in personalised medication and well being fairness.
Key Info:
- GPS integrates genetic research and biobank knowledge to refine illness development predictions.
- The mannequin outperformed 20 different strategies, enhancing prediction accuracy considerably.
- Early identification of at-risk people permits for proactive interventions and therapy.
Supply: Penn State
Autoimmune illnesses, the place the immune system mistakenly assaults the physique’s personal wholesome cells and tissues, typically have a preclinical stage earlier than prognosis that’s characterised by gentle signs or sure antibodies within the blood.
Nevertheless, in some folks, these signs might resolve earlier than culminating within the full illness stage.
Realizing who might progress alongside the illness pathway is important for early prognosis and intervention, improved therapy and higher illness administration, in accordance with a workforce led by researchers from the Penn State School of Drugs that has developed a brand new methodology to foretell the development of autoimmune illness amongst these with preclinical signs.
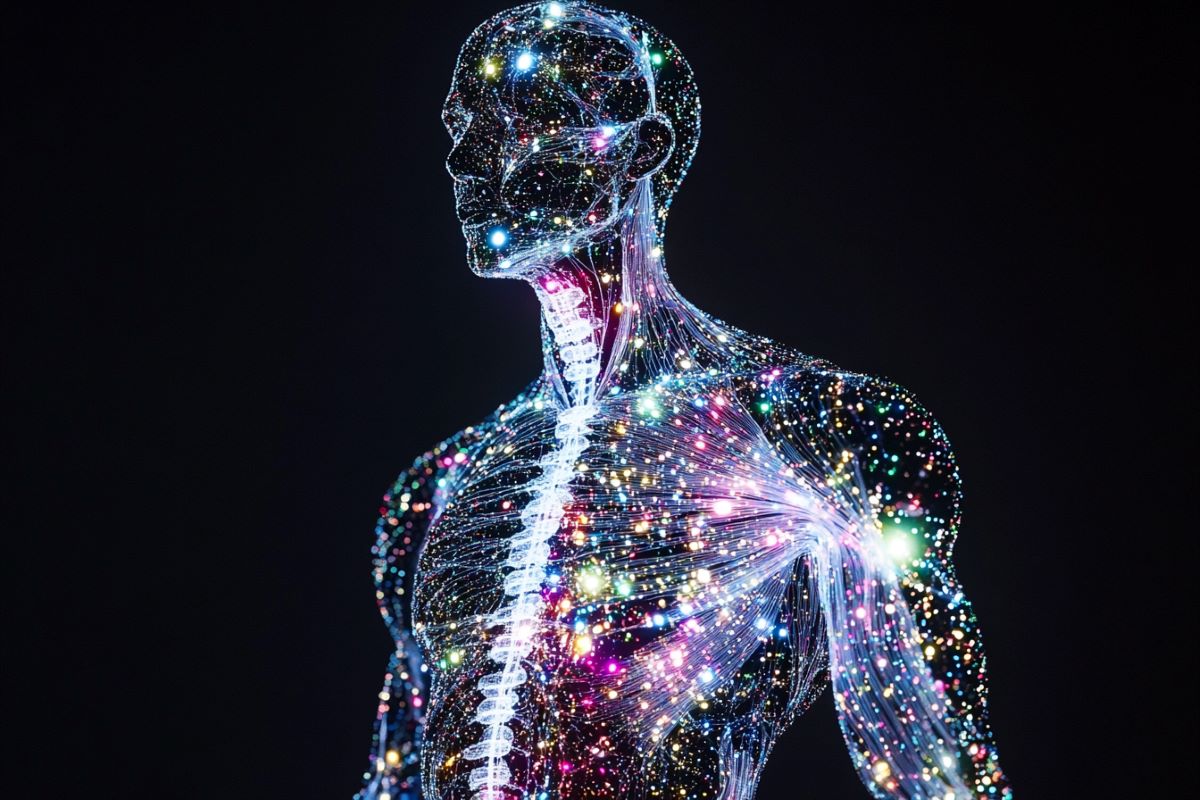
The workforce used synthetic intelligence (AI) to research knowledge from digital well being data and enormous genetic research of individuals with autoimmune illness to provide you with a threat prediction rating.
When in comparison with current fashions, this technique was between 25% and 1,000% extra correct in figuring out whose signs would transfer to superior illness.
The analysis workforce printed their findings this week (Jan. 2) within the journal Nature Communications.
“By focusing on a extra related inhabitants — folks with household historical past or who’re experiencing early signs — we will use machine studying to determine sufferers with the very best threat for illness after which determine appropriate therapeutics that could possibly decelerate the development of the illness.
“It’s much more significant and actionable data,” stated Dajiang Liu, distinguished professor, vice chair for analysis and director of synthetic intelligence and biomedical informatics on the Penn State School of Drugs and co-lead creator of the research.
Roughly 8% of People reside with autoimmune illness, in accordance with the Nationwide Institutes of Health, and the overwhelming majority are ladies. The sooner you’ll be able to detect the illness and intervene, the higher, Liu stated, as a result of as soon as autoimmune illnesses progress, the harm could be irreversible.
There are sometimes indicators of the illness earlier than a person receives a prognosis. For instance, in sufferers with rheumatoid arthritis, antibodies could be detected within the blood 5 years earlier than signs start, the researchers defined.
The problem with forecasting illness development is pattern measurement. The inhabitants of people who’ve a particular autoimmune illness is comparatively small. With much less knowledge out there, it’s tougher to develop an correct mannequin and algorithm, Liu stated.
To enhance prediction accuracy, the analysis workforce developed a brand new methodology, dubbed Genetic Development Rating or GPS, to predict the development from preclinical to illness phases. GPS leverages the concept behind switch studying — a machine studying approach the place a mannequin is educated on one activity or dataset after which fine-tuned for a distinct however associated activity or dataset, defined Bibo Jiang, assistant professor of public well being sciences on the Penn State School of Drugs and lead creator of the research.
It permits researchers to glean higher data from smaller knowledge samples.
For instance, in medical imaging, synthetic intelligence fashions could be educated to inform whether or not a tumor is cancerous or non-cancerous. To create the coaching dataset, medical specialists must label photographs one-by-one, which could be time intensive and restricted by the variety of photographs out there.
Liu stated, as a substitute, switch studying makes use of extra quite a few, easier-to-label photographs, resembling cats and canine and creates a a lot bigger dataset. The duty will also be outsourced. The mannequin learns to distinguish between the animals, after which, it may be refined to differentiate between malignant and benign tumors.
“You don’t want to coach the mannequin from scratch,” Liu stated.
“The way in which that the mannequin segments parts from a picture to find out whether or not it’s a cat or canine is transferable. With some adaptation, you’ll be able to refine the mannequin to separate a picture of a tumor from a picture of regular tissue.”
GPS trains on knowledge from massive case-control genome-wide affiliation research (GWAS), a well-liked strategy in human genetics analysis to determine genetic variations in folks with a particular autoimmune illness from these with out and to detect potential threat components.
It additionally incorporates knowledge from digital well being record-based biobanks, which comprise wealthy details about sufferers, together with genetic variants, lab assessments and medical diagnoses.
This knowledge might help determine people in preclinical phases and characterize the phases of development from preclinical to the illness stage. Information from each sources is then built-in to refine the GPS mannequin, incorporating components which might be related to the precise growth of illness.
“Integrating massive case-control research and biobanks borrowed strengths from the massive pattern sizes of case-control research and improved prediction accuracy,” Liu stated, explaining that folks with excessive GPS scores have the next threat of progressing from preclinical to illness phases.
The workforce used real-world knowledge from the Vanderbilt College biobank to foretell the development of rheumatoid arthritis and lupus after which validated the GPS threat scores with knowledge from the All of Us biobank, a well being knowledge initiative of the Nationwide Institutes of Health.
GPS higher predicted illness development than 20 different fashions that depend on biobank or case-control samples solely and people combining biobank and case-control samples through different strategies.
Correct prediction of illness development utilizing GPS can allow early interventions, focused monitoring and personalised therapy choices, resulting in improved affected person outcomes, Liu stated. It might additionally enhance medical trial design and recruitment by figuring out people who’re most probably to profit from new therapies.
Whereas this research targeted on autoimmune circumstances, the researchers stated {that a} related framework might be used to check different illness varieties.
“Once we discuss underrepresented populations, it’s not nearly race. It is also a gaggle of sufferers which might be under-studied within the medical literature as a result of they comprise solely a small portion of typical knowledge units. AI and switch studying might help us research these populations and assist scale back well being disparities,” Liu stated.
“This work displays the energy of Penn State’s complete analysis program in autoimmune illness.”
Liu and Jiang — together with research co-authors Laura Carrel, professor of biochemistry and molecular biology; Galen Foulke, affiliate professor of dermatology; Nancy Olsen, H. Thomas and Dorothy Willits Hallowell Chair in Rheumatology — shaped the autoimmune working group and have collaborated for almost a decade.
They lead modern medical trials, carry out analysis research to know the organic mechanisms of autoimmune illnesses and develop AI strategies to deal with numerous issues associated to autoimmune illnesses.
Chen Wang, who earned a doctorate in bioinformatics and genomics from Penn State, and Havell Markus, joint diploma pupil within the MD/PhD Medical Scientist Coaching Program, are co-first authors of the research.
Different Penn State authors on the paper embrace: Avantika R. Diwadkar, graduate pupil; Chachrit Khunsriraksakul, who graduated from the MD/PhD Medical Scientist Coaching Program through the time of the research; and Xingyan Wang, who was a analysis assistant at Penn State School of Drugs through the time of the research.
Different contributors embrace Bingshan Li, professor of molecular physiology and biophysics, and Xue Zhong, analysis assistant professor in genetic medication, from Vanderbilt College College of Drugs; and Xiaowei Zhan, affiliate professor of public well being on the College of Texas Southwestern Medical Heart.
Funding: Funding from the Nationwide Institutes of Health, together with the Nationwide Institute of Allergy and Infectious Ailments Workplace of Information Science and Rising Applied sciences, supported this analysis.
About this AI and neurology analysis information
Creator: Christine Yu
Supply: Penn State
Contact: Christine Yu – Penn State
Picture: The picture is credited to Neuroscience Information
Authentic Analysis: Open entry.
“Integrating digital well being data and GWAS abstract statistics to foretell the development of autoimmune illnesses from preclinical phases” by Dajiang Liu et al. Nature Communications
Summary
Integrating digital well being data and GWAS abstract statistics to foretell the development of autoimmune illnesses from preclinical phases
Autoimmune illnesses typically exhibit a preclinical stage earlier than prognosis. Digital well being report (EHR) based-biobanks comprise genetic knowledge and diagnostic data, which might determine preclinical people in danger for development.
Biobanks sometimes have small numbers of circumstances, which aren’t ample to assemble correct polygenic threat scores (PRS). Importantly, development and case-control phenotypes might have shared genetic foundation, which we will exploit to enhance prediction accuracy.
We suggest a novel methodology Genetic Development Rating (GPS) that integrates biobank and case-control research to foretell the illness development threat. Through penalized regression, GPS incorporates PRS weights for case-control research as prior and forces mannequin parameters to be just like the prior if the prior improves prediction accuracy.
In simulations, GPS persistently yields higher prediction accuracy than various methods counting on biobank or case-control samples solely and people combining biobank and case-control samples.
The development is especially evident when biobank pattern is smaller or the genetic correlation is decrease. We derive PRS for the development from preclinical rheumatoid arthritis and systemic lupus erythematosus within the BioVU biobank and validate them in All of Us.
For each illnesses, GPS achieves the very best prediction R2 and the ensuing PRS yields the strongest correlation with development prevalence.
Discussion about this post