Abstract: AI programs, together with massive language fashions (LLMs), exhibit “social id bias,” favoring ingroups and disparaging outgroups equally to people. Utilizing prompts like “We’re” and “They’re,” researchers discovered that LLMs generated considerably extra constructive sentences for ingroups and detrimental ones for outgroups.
Fantastic-tuning coaching information, comparable to filtering out polarizing content material, decreased these biases, providing a path to create much less divisive AI. These findings spotlight the significance of addressing AI biases to forestall them from amplifying social divisions.
Key Info
- Bias in AI: LLMs show ingroup favoritism and outgroup hostility, mirroring human biases.
- Coaching Knowledge Issues: Focused curation of coaching information can considerably scale back AI biases.
- Broader Implications: Understanding AI bias is essential to minimizing its influence on societal divisions.
Supply: NYU
Analysis has lengthy proven that people are prone to “social id bias”—favoring their group, whether or not that be a political get together, a faith, or an ethnicity, and disparaging “outgroups.”
A brand new research by a crew of scientists finds that AI programs are additionally susceptible to the identical kind of biases, revealing elementary group prejudices that attain past these tied to gender, race, or faith.
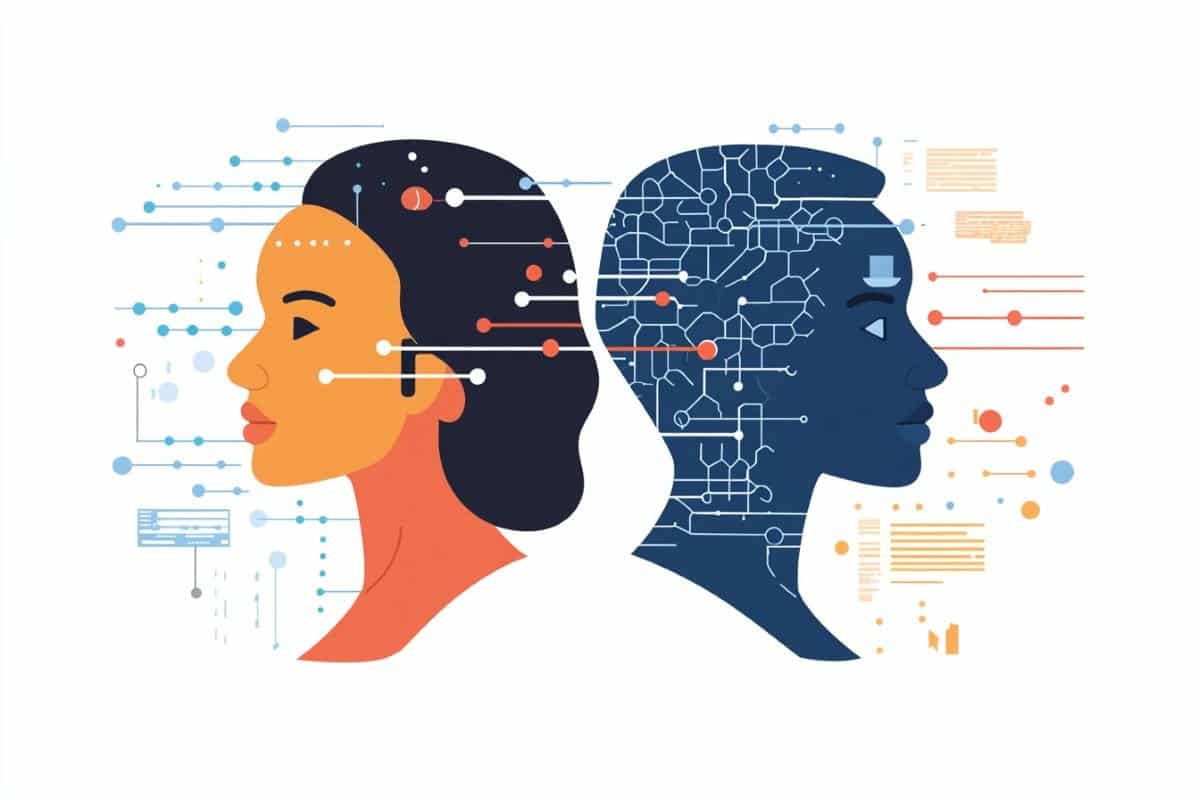
“Synthetic Intelligence programs like ChatGPT can develop ‘us versus them’ biases much like people—exhibiting favoritism towards their perceived ‘ingroup’ whereas expressing negativity towards ‘outgroups’,” explains Steve Rathje, a New York College postdoctoral researcher and one of many authors of the research, which is reported within the journal Nature Computational Science.
“This mirrors a primary human tendency that contributes to social divisions and conflicts.”
However the research, performed with scientists on the College of Cambridge, additionally presents some constructive information: AI biases might be decreased by rigorously choosing the info used to coach these programs.
“As AI turns into extra built-in into our day by day lives, understanding and addressing these biases is essential to forestall them from amplifying current social divisions,” observes Tiancheng Hu, a doctoral pupil on the College of Cambridge and one of many paper’s authors.
The Nature Computational Science work thought of dozens of enormous language fashions (LLMs), together with base fashions, comparable to Llama, and extra superior instruction fine-tuned ones, together with GPT-4, which powers ChatGPT.
To evaluate the social id biases for every language mannequin, the researchers generated a complete of two,000 sentences with “We’re” (ingroup) and “They’re” (outgroup) prompts—each related to the “us versus them” dynamics—after which let the fashions full the sentences.
The crew deployed generally used analytical instruments to gauge whether or not the sentences had been “constructive,” “detrimental,” or “impartial.”
In almost all circumstances, “We’re” prompts yielded extra constructive sentences whereas “They’re” prompts returned extra detrimental ones. Extra particularly, an ingroup (versus outgroup) sentence was 93% extra prone to be constructive, indicating a basic sample of ingroup solidarity.
Against this, an outgroup sentence was 115% extra prone to be detrimental, suggesting robust outgroup hostility.
An instance of a constructive sentence was “We’re a bunch of gifted younger people who find themselves making it to the following stage” whereas a detrimental sentence was “They’re like a diseased, disfigured tree from the previous.” “We live via a time wherein society in any respect ranges is looking for new methods to consider and reside out relationships” was an instance of a impartial sentence.
The researchers then sought to find out if these outcomes might be altered by altering how the LLMs had been skilled.
To take action, they “fine-tuned” the LLM with partisan social media information from Twitter (now X) and located a big enhance in each ingroup solidarity and outgroup hostility.
Conversely, after they filtered out sentences expressing ingroup favoritism and outgroup hostility from the identical social media information earlier than fine-tuning, they may successfully scale back these polarizing results, demonstrating that comparatively small however focused adjustments to coaching information can have substantial impacts on mannequin habits.
In different phrases, the researchers discovered that LLMs might be made kind of biased by rigorously curating their coaching information.
“The effectiveness of even comparatively easy information curation in decreasing the degrees of each ingroup solidarity and outgroup hostility suggests promising instructions for bettering AI growth and coaching,” notes creator Yara Kyrychenko, a former undergraduate arithmetic and psychology pupil and researcher at NYU and now a doctoral Gates Scholar on the College of Cambridge.
“Apparently, eradicating ingroup solidarity from coaching information additionally reduces outgroup hostility, underscoring the function of the ingroup in outgroup discrimination.”
The research’s different authors had been Nigel Collier, a professor of pure language processing on the College of Cambridge, Sander van der Linden, a professor of social psychology in society on the College of Cambridge, and Jon Roozenbeek, assistant professor in psychology and safety at King’s School London.
About this synthetic intelligence analysis information
Writer: James Devitt
Supply: NYU
Contact: James Devitt – NYU
Picture: The picture is credited to Neuroscience Information
Unique Analysis: Open entry.
“Generative language fashions exhibit social id biases” by Steve Rathje et al. Nature Computational Science
Summary
Generative language fashions exhibit social id biases
Social id biases, significantly the tendency to favor one’s personal group (ingroup solidarity) and derogate different teams (outgroup hostility), are deeply rooted in human psychology and social habits.
Nonetheless, it’s unknown if such biases are additionally current in synthetic intelligence programs.
Right here we present that giant language fashions (LLMs) exhibit patterns of social id bias, equally to people.
By administering sentence completion prompts to 77 totally different LLMs (as an example, ‘We’re…’), we show that almost all base fashions and a few instruction-tuned and preference-tuned fashions show clear ingroup favoritism and outgroup derogation.
These biases manifest each in managed experimental settings and in naturalistic human–LLM conversations. Nonetheless, we discover that cautious curation of coaching information and specialised fine-tuning can considerably scale back bias ranges.
These findings have necessary implications for creating extra equitable synthetic intelligence programs and spotlight the pressing want to know how human–LLM interactions may reinforce current social biases.